Recommendation Systems in Diagnosis: Learning Models for Clinical Personalization and Diagnostic Algorithms
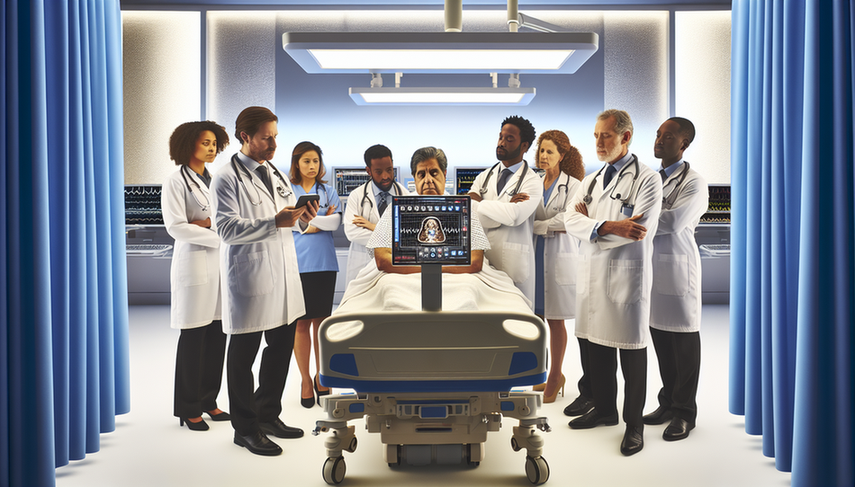
In the era of digital medicine, recommendation systems are transforming the way we approach clinical diagnosis. These systems, powered by learning models, enable a personalization of diagnosis that adapts to the individual characteristics of each patient. The integration of advanced clinical algorithms into daily medical practice not only enhances diagnostic accuracy but also optimizes time and resources, allowing physicians to focus on critical decision-making.
Diving Deeper into Learning Models for Clinical Personalization
Learning models have proven to be powerful tools in modern medicine. The ability of these models to analyze large volumes of data and extract complex patterns is fundamental for the development of effective recommendation systems. A notable example is the use of machine learning in medicine, which has enabled significant advances in computer-assisted diagnosis and personalized medicine.
The integration of artificial intelligence in clinical decision support systems is revolutionizing medical practice. These systems utilize algorithms such as neural networks and decision trees to provide personalized treatment recommendations, risk predictions, and AI-assisted clinical documentation. Furthermore, the transformation of artificial intelligence in the future of healthcare is enabling greater learning capacity and support at scale, changing the way clinical decisions are made.
In the field of tumor pathology, algorithms based on deep learning are improving diagnostic accuracy and reducing the workload of pathologists, allowing them to dedicate more time to high-level decision-making tasks. These advances are crucial for meeting the requirements of precision oncology, where treatment personalization is essential.
Conclusions
The implementation of recommendation systems based on learning models is redefining the landscape of clinical diagnosis. These systems not only enhance the accuracy and efficiency of diagnosis but also enable more personalized and patient-centered care. As we continue to advance in the integration of these technologies, it is essential to address the ethical and implementation challenges to maximize their potential in daily clinical practice.
Referencias
- [1] eDoctor: machine learning and the future of medicine
- [2] AI-Driven Clinical Decision Support Systems: An Ongoing Pursuit of Potential
- [3] Artificial Intelligence Transforms the Future of Health Care
- [4] Emerging role of deep learning-based artificial intelligence in tumor pathology
Created 24/1/2025