Clinical Data Mining: Information Processing for Evidence-Based Diagnosis Using Machine Learning
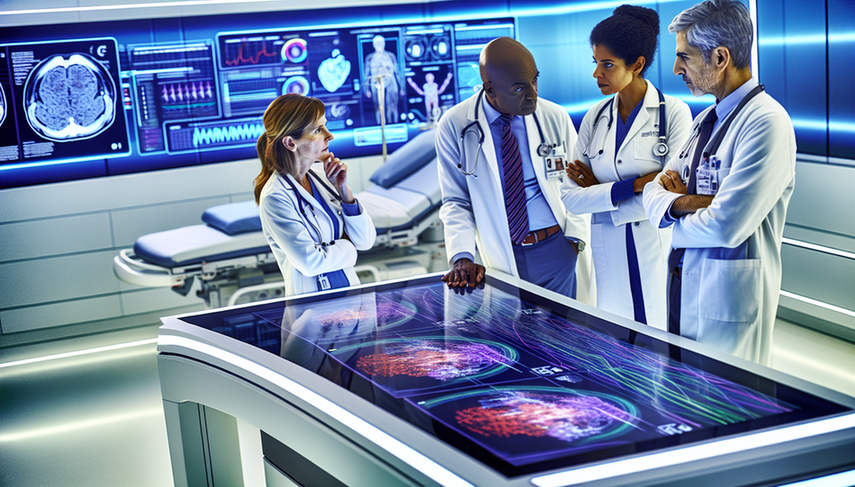
In the information age, clinical data mining has become an essential tool for information processing that enables healthcare professionals to perform evidence-based diagnosis. The integration of advanced technologies such as machine learning and artificial intelligence is transforming the way clinical data is analyzed and utilized, allowing for more precise and personalized medical care.
Diving Deeper into Clinical Data Mining
Clinical data mining involves the extraction and analysis of large volumes of medical data to identify patterns and relationships that may not be immediately apparent. An example of this is the use of integrative databases like SymMap, which combines traditional Chinese medicine with modern medicine to enhance drug discovery and clinical diagnosis. This database allows for the mapping of symptoms and their relationships with herbs and diseases, providing a platform for evidence-based drug discovery.
Moreover, the use of advanced algorithms such as the FP-Growth algorithm within the Hadoop framework has proven effective in analyzing the compositions of traditional Chinese medicine, improving accuracy and retrieval rates compared to traditional methods. This approach facilitates the discovery of rules for typical clinical symptoms and treatment plans, enabling an evidence-based medical evaluation.
In the field of oncology, the integration of artificial intelligence in cancer genomics is revolutionizing precision medicine. The ability to process large volumes of genetic data and transform them into actionable clinical knowledge is significantly enhancing cancer care, although challenges remain regarding algorithmic transparency and reproducibility.
Conclusions
Clinical data mining and information processing are fundamental to advancing towards evidence-based diagnosis. The integration of technologies such as machine learning and artificial intelligence is enabling physicians to make more informed and personalized decisions, thereby improving the quality of medical care. However, it is crucial to address the challenges associated with the adoption of these technologies to ensure their safety and efficacy in the clinical environment.
Referencias
- [1] SymMap: an integrative database of traditional Chinese medicine enhanced by symptom mapping.
- [2] TCM Constitution Analysis Method Based on Parallel FP-Growth Algorithm in Hadoop Framework.
- [3] Translating cancer genomics into precision medicine with artificial intelligence: applications, challenges and future perspectives.
Created 24/1/2025