Fracture Detection Using Deep Learning: Advances in Early Diagnosis with Radiological Imaging Assistance
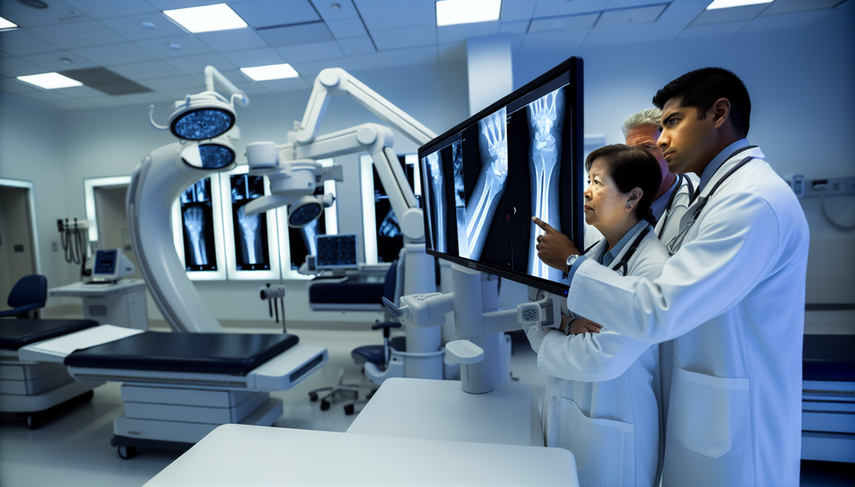
The detection of fractures is a crucial component in the management of patients with bone injuries. Traditionally, diagnosis has relied on the interpretation of medical images by experienced radiologists. However, with advancements in technology, radiological deep learning has emerged as a powerful tool to enhance the accuracy and speed of early diagnosis of fractures. This approach not only optimizes diagnostic time but also reduces the risk of human error, allowing for more timely and effective intervention.
Advances in Fracture Detection with Deep Learning
The use of deep learning in fracture detection has shown promising results across various areas of the body. For instance, a recent study developed a model based on Mask R-CNN for the detection of vertebral compression fractures, achieving high accuracy and sensitivity in identifying fractures in plain radiographs. This type of medical imaging assistance enables physicians to make more informed decisions in the initial diagnosis.
Another significant advancement has been observed in the detection of scaphoid fractures using artificial intelligence. A meta-analysis recently demonstrated that AI systems can achieve high sensitivity and specificity, which is crucial given that these fractures are often overlooked in conventional radiographs.
Furthermore, the application of deep neural networks in the detection of femoral neck fractures has proven to be superior to traditional methods. A study utilizing the DAFDNet method demonstrated an accuracy exceeding 94.8% in identifying non-displaced fractures, surpassing even diagnoses made by experienced orthopedic surgeons.
Conclusions
The integration of bone patterns and deep learning algorithms in medical diagnosis is transforming the way fractures are detected. These advancements not only improve diagnostic accuracy but also facilitate quicker and more effective intervention, reducing the risk of long-term complications. As technology continues to evolve, we are likely to see even greater adoption of these tools in daily clinical practice, thereby enhancing patient outcomes.
Referencias
- [1] Deep learning application of vertebral compression fracture detection using mask R-CNN
- [2] Artificial intelligence for X-ray scaphoid fracture detection: a systematic review and diagnostic test accuracy meta-analysis
- [3] A Computer-Assisted Diagnostic Method for Accurate Detection of Early Nondisplaced Fractures of the Femoral Neck
Created 20/1/2025