AI in MRI: Automatic Segmentation Algorithms and New Diagnostic Horizons in Magnetic Resonance Imaging
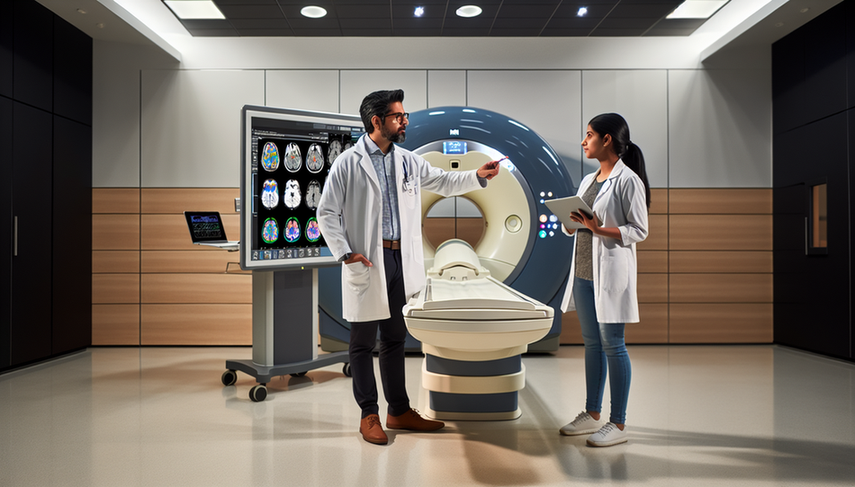
Magnetic resonance imaging (MRI) has revolutionized medical diagnostics by providing detailed images of the soft tissues in the human body. However, the interpretation of these images can be complex and requires a high level of expertise. In this context, artificial intelligence (AI) has emerged as a powerful tool to enhance the accuracy and efficiency of automatic segmentation in MRI, opening new horizons in medical diagnostics.
Advancements in Automatic Segmentation with AI
AI in MRI has proven particularly useful in image segmentation, a crucial process for identifying and delineating anatomical structures and lesions. Medical algorithms based on deep learning, such as convolutional neural networks, have shown remarkable precision in segmenting tissues across various pathologies. For instance, in the case of breast cancer, AI has improved lesion segmentation and biological characterization of cancer, facilitating more accurate diagnoses and better treatment planning [1].
In the realm of multiparametric magnetic resonance imaging (mpMRI), AI has been employed to classify, segment, and detect diseases, enhancing treatment planning and predicting clinical outcomes [2]. Additionally, in the diagnosis of multiple sclerosis, AI models have been extensively applied for lesion segmentation and biomarker identification, allowing for better monitoring and management of the disease [3].
Conclusions and Future of AI in Magnetic Resonance Imaging
The integration of AI in magnetic resonance imaging is transforming clinical practice by providing tools that enhance diagnostic accuracy and optimize interpretation time. However, for these technologies to reach their full potential, it is crucial to address challenges such as model interpretability and the need for larger and more diverse datasets. As AI continues to evolve, its application in MRI is expected not only to improve automatic segmentation but also to facilitate personalized treatments and enhance patient outcomes.
Referencias
- [1] Recent advancements in artificial intelligence for breast cancer: Image augmentation, segmentation, diagnosis, and prognosis approaches
- [2] Artificial intelligence in multiparametric magnetic resonance imaging: A review
- [3] Artificial Intelligence and Multiple Sclerosis
Created 20/1/2025