Tumor Classification with Machine Learning: Advancing Personalized Diagnosis through Molecular Analysis and Biomarkers
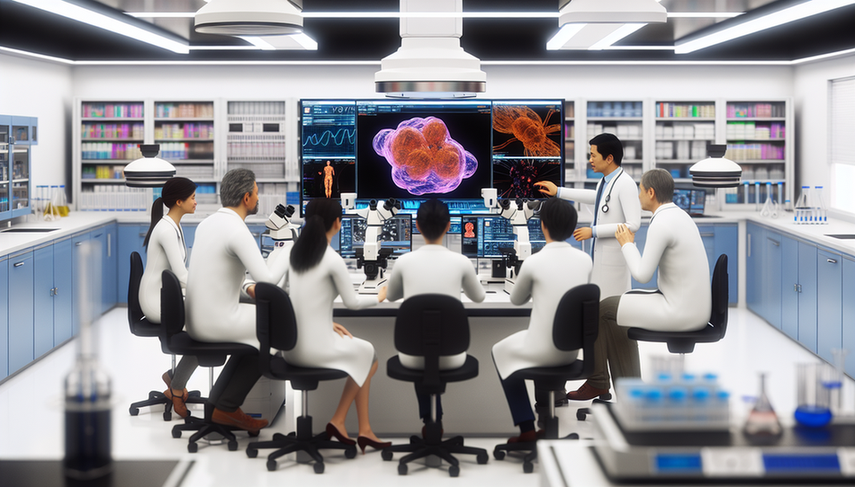
The tumor classification has significantly evolved with the advancement of artificial intelligence (AI) and machine learning. These technologies are transforming medical diagnosis, enabling a more precise and personalized approach based on tumor biomarkers. The integration of these tools into clinical practice promises to enhance diagnostic accuracy and patient prognosis, facilitating a personalized diagnosis that adapts to the specific molecular characteristics of each tumor.
Diving into Molecular Analysis and Machine Learning
The use of AI in oncology has focused on developing models that can analyze large volumes of imaging and genomic data to identify patterns that are not evident at first glance. For instance, in the field of neuro-oncology, imaging biomarkers are being utilized to provide objective information about tumor biology and treatment response. These advancements allow for a better understanding of the tumor microenvironment and immune response, which is crucial for the development of targeted therapies.
In the realm of gastrointestinal cancers, AI-based diagnostic support systems, particularly convolutional neural networks, have shown great potential in characterizing and prognosticating cancer pathology. However, large-scale trials are needed to evaluate their performance and clinical utility.
Moreover, in prostate cancer, epithelial cell marker genes have been identified that improve outcomes and immunotherapy, bringing us closer to personalized therapeutic approaches. These advancements underscore the importance of integrating multi-omic data and AI models to enhance diagnostic and treatment precision.
Conclusions
The application of AI in tumor classification and molecular analysis is revolutionizing oncological diagnosis. By enabling a more detailed and personalized approach, these technologies are paving the way for a personalized diagnosis based on tumor biomarkers. As we continue to develop and validate these tools, it is essential for physicians to stay informed about these advancements to effectively integrate these innovations into daily clinical practice.
Referencias
- [1] Imaging biomarkers for clinical applications in neuro-oncology: current status and future perspectives
- [2] Gastrointestinal cancer classification and prognostication from histology using deep learning: Systematic review
- [3] Integrated machine learning identifies epithelial cell marker genes for improving outcomes and immunotherapy in prostate cancer
Created 20/1/2025