AI-Assisted Parkinson's Diagnosis: Enhancing Clinical Algorithms and Monitoring Systems in Neurological Telemedicine
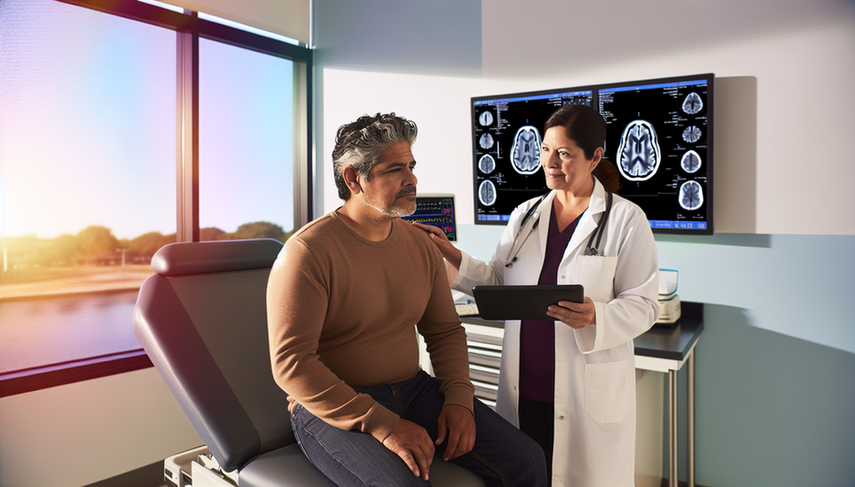
The diagnosis of Parkinson's has traditionally posed challenges due to symptom variability and the absence of precise biomarkers. However, the integration of artificial intelligence (AI) in medicine is revolutionizing our approach to movement disorders. AI is not only enhancing diagnostic accuracy but also facilitating neurological telemedicine and continuous patient monitoring systems.
AI in Parkinson's Diagnosis
AI and machine learning are being utilized to identify patterns in complex data that are difficult to detect through traditional methods. For instance, AI models have been developed to analyze nocturnal breathing signals to detect Parkinson's disease with remarkable accuracy, enabling non-invasive home assessments (see study). Additionally, the use of liquid biopsies combined with AI has allowed for the identification of specific protein markers that may indicate the presence of Parkinson's, even before symptoms become evident (see study).
Another significant advancement is the use of EEG signals and deep learning techniques to classify and diagnose Parkinson's. These techniques have proven to be highly effective, achieving accuracies of up to 99% in patient classification (see study). Furthermore, AI is being employed to analyze fMRI data and predict optimal deep brain stimulation configurations, which could significantly enhance patient treatment (see study).
Conclusions
The integration of AI in the diagnosis and management of Parkinson's is transforming the field of clinical algorithms and monitoring systems. These advancements not only improve diagnostic precision but also enable closer and more personalized patient follow-up, facilitating neurological telemedicine. As technology continues to advance, we are likely to see greater adoption of these tools in daily clinical practice, thereby enhancing the quality of life for patients with Parkinson's.
Referencias
- [1] Artificial intelligence-enabled detection and assessment of Parkinson's disease using nocturnal breathing signals.
- [2] Liquid-biopsy proteomics combined with AI identifies cellular drivers of eye aging and disease in vivo.
- [3] Diagnosis and classification of Parkinson's disease using ensemble learning and 1D-PDCovNN.
- [4] Predicting optimal deep brain stimulation parameters for Parkinson's disease using functional MRI and machine learning.
Created 20/1/2025