Multicenter Collaboration and AI: Advancing Global Genetic Research through Collaborative Studies and Big Data
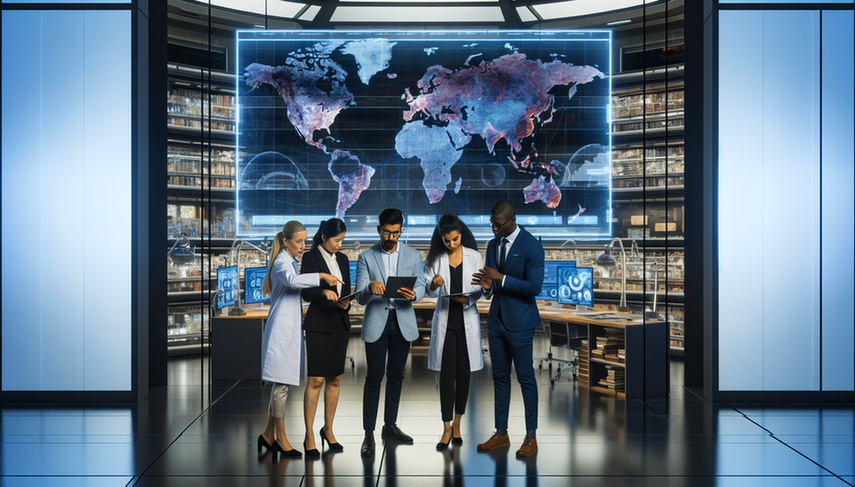
In the era of multicenter collaboration and technological advancement, artificial intelligence (AI) has become a fundamental pillar for global genetic research. The ability to integrate data from multiple research centers and apply advanced algorithms has enabled unprecedented progress in understanding complex diseases and developing personalized therapies. This approach not only enhances the accuracy of studies but also accelerates the discovery of new scientific knowledge.
Diving into Multicenter Collaboration and AI in Genetics
AI in genetics has proven to be a powerful tool for analyzing large volumes of genetic and clinical data. An example of this is the multicenter study investigating the prognostic value of spatial features from brain MRI in diffuse gliomas, utilizing a three-dimensional convolutional neural network. This study, which included data from five different datasets, demonstrated that the combination of clinical, genetic, and imaging data provides the best performance in predicting patient survival [1].
Another example is the Discovery BPD program, which employs a collaborative approach to investigate bronchopulmonary dysplasia in very low birth weight newborns. This program integrates detailed clinical data analysis through machine learning, genetic susceptibility studies, and molecular translation, representing a significant advancement in understanding this complex disease [2].
Furthermore, the creation of public databases, such as the preterm birth genetics knowledge base (PTBGene), fosters collaboration among research networks by providing shared access to genetic data, facilitating the identification of genetic associations with preterm birth [3].
Conclusions
The integration of AI in genetics and multicenter collaboration is transforming medical research, allowing for a more precise and efficient approach to studying complex diseases. The ability to share and analyze global big data through research networks not only accelerates scientific discovery but also enhances the capacity to develop personalized treatments. As we continue to advance in this direction, it is essential to maintain an ethical and collaborative approach that ensures public trust in our work.
Referencias
- [1] Added prognostic value of 3D deep learning-derived features from preoperative MRI for adult-type diffuse gliomas
- [2] The discovery BPD (D-BPD) program: study protocol of a prospective translational multicenter collaborative study to investigate determinants of chronic lung disease in very low birth weight infants
- [3] Synopsis of preterm birth genetic association studies: the preterm birth genetics knowledge base (PTBGene)
Created 20/1/2025