Model Transparency and Explainability: Building Trust in AI for Medical Decision-Making and Professional Accountability
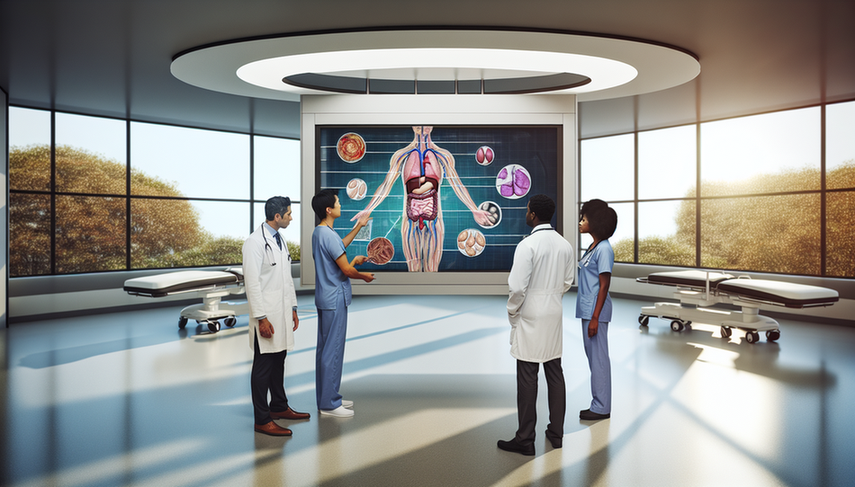
The artificial intelligence (AI) is transforming the field of medicine, offering advanced tools for diagnosis and clinical decision-making. However, the "black box" nature of many AI models poses significant challenges in terms of model transparency and trust in AI. The ability to explain how and why an AI model arrives at a decision is crucial for its acceptance and use in clinical settings. This concept, known as explainability, is fundamental to ensuring professional accountability and patient safety.
Diving Deeper into Explainability and Trust
Explainability in medical AI refers to the ability of AI systems to provide clear and understandable reasoning behind their predictions or decisions. This is especially important in areas such as medical imaging, where the interpretation of complex images can directly influence patient treatment. However, current methods of explainability are not yet mature enough for clinical implementation, as the explanations they provide can be difficult for medical experts to comprehend.
Trust in AI systems also depends on the quality of training data and the model's ability to generalize its results to different clinical contexts. A recent study on ethical implications of AI in healthcare highlights the importance of transparency and accountability in AI decision-making processes to enhance trust and professional accountability.
Moreover, physician understanding and trust in AI outcomes are essential for its adoption. Physicians prefer AI results accompanied by model-agnostic explanations, even though the method of explainability does not significantly alter the expected behavior of the physician.
Conclusions
The integration of AI in medicine offers unprecedented opportunities to improve medical decision-making. However, for these technologies to be effective and safe, it is crucial to address challenges related to model transparency and trust in AI. Explainability not only enhances the understanding of AI systems but also strengthens the trust of medical professionals in these tools, ensuring they are used responsibly and ethically. As research progresses, it is essential to develop more comprehensible and effective explainability methods to facilitate the adoption of AI in clinical practice.
Referencias
- [1] Current status and future directions of explainable artificial intelligence in medical imaging.
- [2] Ethical implications of AI and robotics in healthcare: A review.
- [3] Physician understanding, explainability, and trust in a hypothetical machine learning risk calculator.
Created 20/1/2025