Machine Learning vs Deep Learning: Key Differences and Applications in Digital Clinical Practice
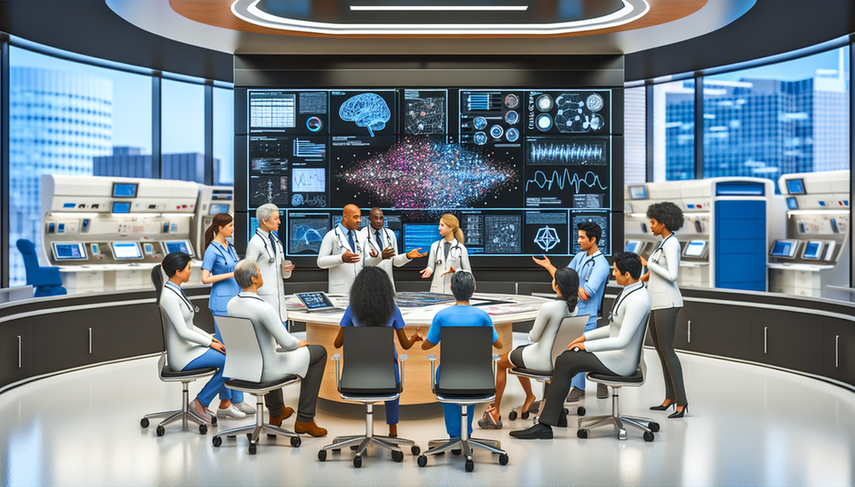
In recent years, artificial intelligence (AI) has revolutionized the field of medicine, particularly through machine learning in healthcare and clinical deep learning. These advancements have enabled the development of medical algorithms that enhance diagnostic accuracy and optimize digital clinical practice. However, it is crucial to understand the differences between these two technologies and how they are applied in the clinical environment.
Diving Deeper into Machine Learning and Deep Learning
Machine learning in healthcare refers to algorithms that learn from data to make predictions or decisions without being explicitly programmed for each task. It is widely used in areas such as anesthesiology, where it assists in monitoring anesthesia depth and predicting events and risks.
On the other hand, clinical deep learning is a subcategory of machine learning that utilizes deep neural networks to analyze large volumes of complex data. It has proven particularly effective in medical image analysis, such as in radiology, where it outperforms conventional techniques in pattern recognition tasks.
A notable example of deep learning in clinical practice is its application in thoracic oncology, where it is used for the detection and characterization of pulmonary nodules. Additionally, in the field of radiomics, deep learning enables the extraction and analysis of quantitative data from medical images, improving accuracy in cancer detection and prognosis.
Conclusions
The integration of machine learning and deep learning in clinical practice is transforming modern medicine. Although both approaches have their own strengths and limitations, their combined application promises to significantly enhance diagnostic accuracy and treatment efficiency. However, it is essential to address challenges such as model interpretability and clinical validation to maximize their impact on patient care.
Referencias
- [1] Artificial Intelligence in Anesthesiology: Current Techniques, Clinical Applications, and Limitations
- [2] Artificial intelligence in radiology
- [3] Artificial intelligence in lung cancer: current applications and perspectives
- [4] A deep look into radiomics
Created 20/1/2025