AI for Assessing Coronary Risk: Clinical Algorithms for Heart Attack Prediction and Primary Prevention for a Healthy Heart
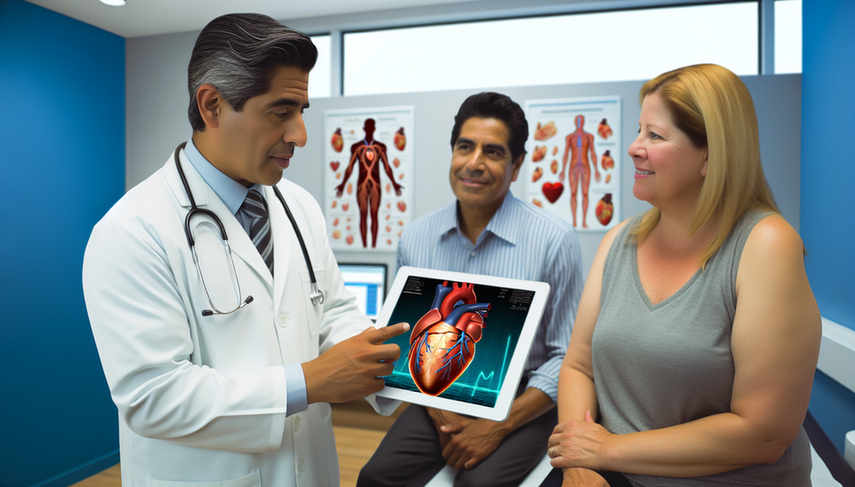
The primary prevention of cardiovascular diseases, particularly heart attacks, remains a constant challenge in medical practice. Traditionally, clinical algorithms have been the primary tool for assessing coronary risk. However, these methods often fail to capture the complexity of individual risk factors. In this context, artificial intelligence (AI) emerges as a promising tool to enhance heart attack prediction and optimize prevention strategies for a healthy heart.
Advances in AI for Coronary Risk Assessment
The application of AI in cardiovascular medicine has enabled the development of more accurate models for risk assessment. A recent study highlights how AI can integrate multiple risk factors, thereby improving the accuracy of models predicting major adverse cardiovascular events (MACE) (see study). Additionally, the combination of electrocardiogram (ECG) data with coronary calcium scores has proven effective in identifying at-risk populations (see study).
Another innovative approach is the use of biomarkers derived from purine metabolites, which have shown potential in predicting and diagnosing coronary disease through machine learning models (see study). These advancements underscore AI's ability to integrate complex data and provide a more personalized assessment of coronary risk.
Conclusions and Future Perspectives
The integration of AI into clinical practice for assessing coronary risk and primary prevention of heart attacks represents a significant shift towards a more personalized and precise approach. AI models not only enhance the accuracy of predictions but also allow for the identification of non-traditional risk factors that may be overlooked by conventional methods (see study). As we continue to explore these technologies, it is crucial to validate these models in diverse populations to ensure their applicability and effectiveness in daily clinical practice.
References
- [1] Machine learning derived ECG risk score improves cardiovascular risk assessment in conjunction with coronary artery calcium scoring.
- [2] Purine metabolite-based machine learning models for risk prediction, prognosis, and diagnosis of coronary artery disease.
- [3] Using machine learning to predict acute myocardial infarction and ischemic heart disease in primary care cardiovascular patients.
Created 20/1/2025