Algorithmic Biases in Medical Diagnosis: Ensuring Health Equity and Ethical AI Practices
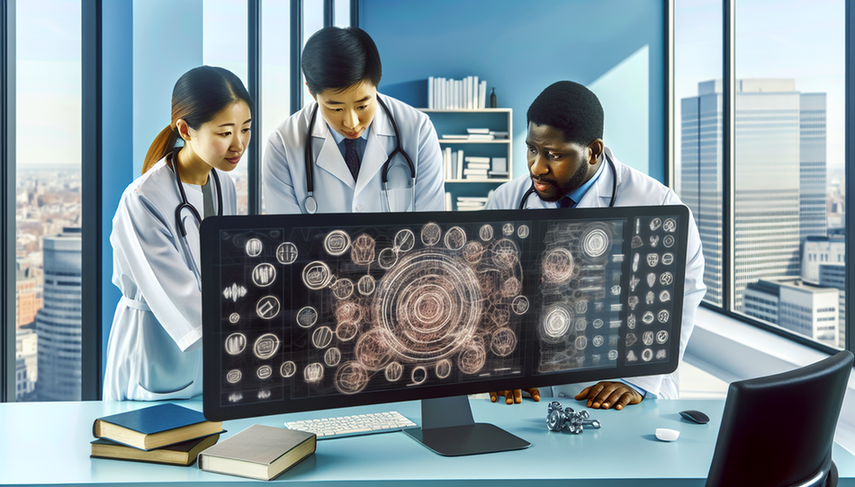
Artificial intelligence (AI) and machine learning are transforming the field of medical diagnosis, offering the promise of improved accuracy and efficiency in healthcare. However, the implementation of these technologies is not without challenges, particularly concerning algorithmic biases that can lead to unequal diagnosis and compromise health equity. This article explores how these biases can arise and what measures can be taken to ensure that AI is used ethically and equitably.
Understanding Algorithmic Biases in Medical Diagnosis
Algorithmic biases in medical diagnosis can stem from various sources, including the lack of diversity in the datasets used to train AI models. For instance, a study on the application of AI in gastroenterology and hepatology highlights how the failure to recognize these biases can exacerbate racial, ethnic, and gender disparities in the diagnosis and treatment of diseases such as esophageal cancer and inflammatory bowel disease.
In the field of dermatology, it has been observed that AI algorithms can introduce biased machine learning if the training data does not adequately represent all gender and ethnic groups. An article on gender equity in AI applications in dermatology underscores the importance of considering sex and gender differences in the development of these tools to avoid undesirable biases.
Moreover, in radiology, the lack of diversity in datasets can lead to biased outcomes. A study on biases in medical imaging emphasizes how AI algorithms can either improve or perpetuate existing biases, depending on how they are designed and applied.
Conclusions and Recommendations for Ensuring Health Equity in AI
To address algorithmic biases and promote health equity, it is crucial to adopt an ethical and transparent approach in the development and implementation of AI technologies. This includes creating diverse and representative datasets, as well as external validation of AI models in diverse populations. An article on considerations for addressing bias in AI proposes a product lifecycle framework that spans from design to implementation, ensuring that biases are addressed at every phase.
Furthermore, it is essential for healthcare professionals to be trained to understand and mitigate biases in AI models. Interdisciplinary education and collaboration among clinicians, researchers, and AI developers are vital to ensure that these technologies are used in ways that benefit all patients equally.
Referencias
- [1] Artificial intelligence in gastroenterology and hepatology: how to advance clinical practice while ensuring health equity.
- [2] Towards gender equity in artificial intelligence and machine learning applications in dermatology.
- [3] Machine Learning and Bias in Medical Imaging: Opportunities and Challenges.
- [4] Considerations for addressing bias in artificial intelligence for health equity.
Created 20/1/2025