AI in Pulmonology: Early Detection of Respiratory Diseases Using Deep Learning Techniques
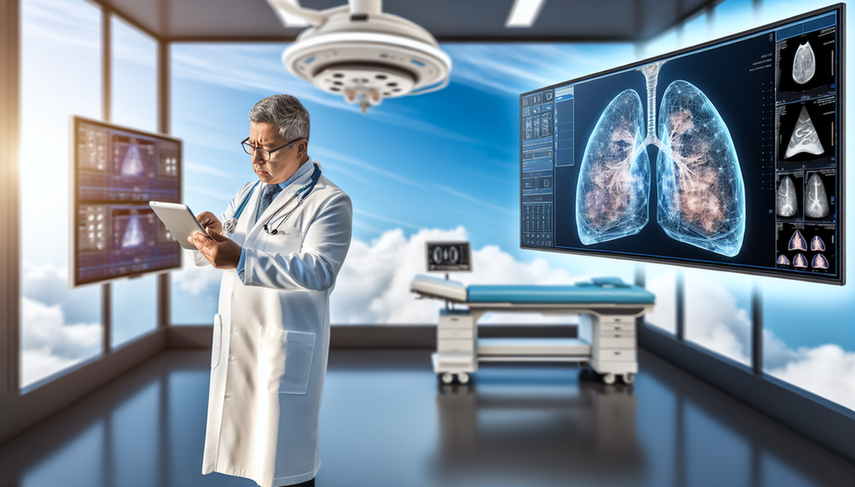
Artificial Intelligence (AI) has revolutionized numerous fields of medicine, and pulmonology is no exception. In particular, the use of deep learning has enabled significant advancements in the diagnosis of respiratory diseases, facilitating the early detection of conditions that might otherwise go unnoticed until more advanced stages. This article explores how these technologies are transforming clinical practice and improving patient outcomes.
Advancements in Early Detection of Respiratory Diseases
Deep learning has proven to be a powerful tool in the detection of pulmonary nodules and in the early characterization of lung cancer. A recent study highlighted how advanced image analysis models can enhance the classification of nodules and the early characterization of lung cancer, surpassing traditional methods in accuracy and efficiency [1].
Moreover, the integration of genomic features and the use of blood biomarkers have shown significant potential for non-invasive early lung cancer detection [2]. These approaches not only improve diagnostic accuracy but also allow for more timely intervention, which is crucial for enhancing survival rates.
In the realm of interstitial lung diseases, machine learning has been applied to identify imaging biomarkers that can predict the extent of pulmonary fibrosis and correlate radiological abnormalities with the decline in pulmonary function [3]. These advancements underscore the potential of AI to bridge gaps in the diagnosis and prognosis of these complex diseases.
Conclusions
The application of AI in pulmonology is transforming the landscape of respiratory disease diagnosis. The use of deep learning not only enhances the early detection of conditions such as lung cancer and interstitial diseases but also offers new opportunities for more precise and personalized interventions. As these technologies continue to evolve, it is essential for healthcare professionals to stay informed and prepared to integrate these tools into daily clinical practice, thereby improving outcomes for patients.
References
- [1] Expanding Role of Advanced Image Analysis in CT-detected Indeterminate Pulmonary Nodules and Early Lung Cancer Characterization.
- [2] Integrating genomic features for non-invasive early lung cancer detection.
- [3] Machine learning in radiology: the new frontier in interstitial lung diseases.
Created 24/1/2025