AI in Gynecology: Smart Ultrasounds and Clinical Algorithms for Fetal Anomaly Detection
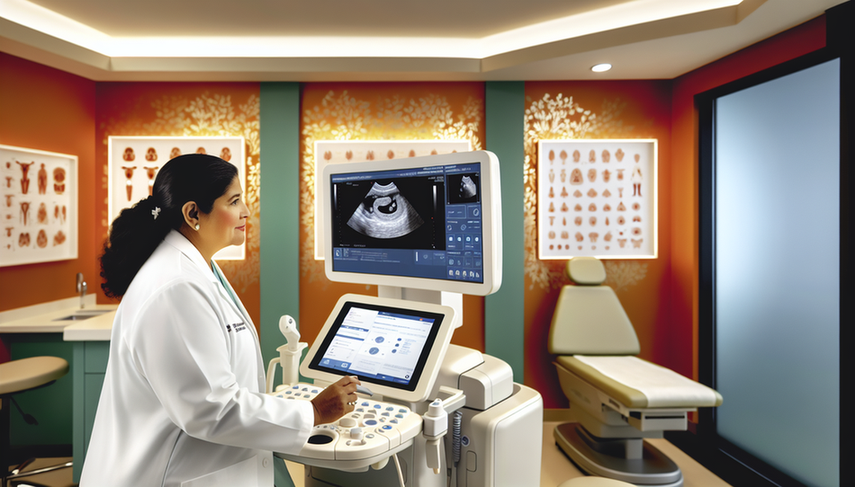
The artificial intelligence (AI) is revolutionizing the field of gynecology, particularly in the realm of smart ultrasounds for fetal anomaly detection. The ability of AI algorithms to analyze ultrasound images with precision and speed is transforming how physicians approach prenatal diagnosis. This advancement not only enhances diagnostic accuracy but also optimizes time and resources in gynecology clinics.
Advances in Fetal Anomaly Detection Using AI
The implementation of AI in ultrasounds has enabled significant advancements in the detection of fetal anomalies. A recent study has demonstrated that deep learning models can outperform traditional clinical detection of congenital heart defects, even in images acquired in low-risk community settings. These models not only improve sensitivity but also offer remarkable specificity, which is crucial for reducing false positives.
Moreover, AI has proven effective in the classification of fetal heart ultrasound images, identifying ventricular septal defects with impressive accuracy. This type of technology is especially beneficial for less experienced physicians, as it provides a reliable and rapid second opinion.
Another significant advancement is the use of AI for detection of fetal intracranial anomalies. A recently developed AI system has demonstrated performance comparable to that of experts in identifying abnormal intracranial image patterns, underscoring its potential as an effective screening tool in clinical practice.
Conclusions
The integration of AI in gynecology and smart ultrasounds is redefining the standard of care in fetal anomaly detection. These clinical algorithms not only enhance diagnostic accuracy but also optimize resource and time utilization in clinics. As technology continues to advance, we are likely to see even greater adoption of these tools in daily clinical practice, thereby improving outcomes for mothers and their future children.
Referencias
- [1] Deep-learning model for prenatal congenital heart disease screening generalizes to community setting and outperforms clinical detection.
- [2] Classification of normal and abnormal fetal heart ultrasound images and identification of ventricular septal defects based on deep learning.
- [3] Use of real-time artificial intelligence in detection of abnormal image patterns in standard sonographic reference planes in screening for fetal intracranial malformations.
Created 24/1/2025